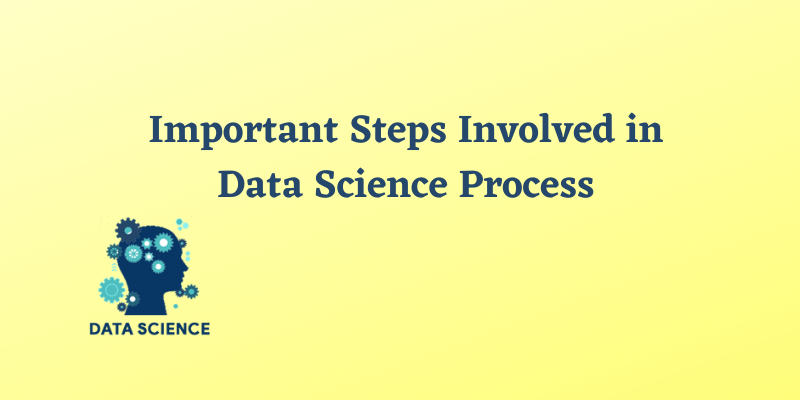
Data science is a discipline of study that combines subject-matter knowledge, programming abilities, and competence in math and statistics to draw forth essential insights from data. Data scientists use machine learning algorithms on various data types, including numbers, text, photos, video, and audio, to create artificial intelligence (AI) systems. Here we discuss the Important Steps involved in Data Science Process, and if you want to know more about Data Science, Join Data Science Course in Chennai at FITA Academy.
Steps involved in Data Science Process:
Lifecycle indicates the iterative processes necessary to conceive, manufacture, and maintain any data science product. The life cycle of every project is unique, just like how they are each generated. An extensive life cycle that includes some of the most common data science operations is still conceivable.
Framing the problem:
Understanding and framing the problem is the initial stage of the data science life cycle. Then, using this framework, you can develop a potent valuable model for your business.
Enroll Data Science Online Course, where the learners can learn about Data Science at their flexible time.
Collection:
Next, the correct collection of It needs to be gathered. It is imperative to have access to high-quality, focused data and the resources to collect it to achieve meaningful results. Given that a sizable percentage of the 2.5 quintillion bytes of data produced daily is in unstructured formats, you’ll likely need to extract the data and convert it into a usable format, such as a CSV or JSON file, next.
Cleaning:
Most of the data you get throughout the collecting phase will be unstructured, unrelated, and unfiltered. The quality of your data will greatly impact your analysis’ accuracy and efficacy because adequate data leads to accurate conclusions. With the greatest data science education and resources accessible, it is simple to learn.
When data is cleaned, duplicate and null values, damaged, mismatched kinds, inaccurate entries, missing data, and improper formatting are all eliminated. The most time-consuming phase of producing models that work is finding and addressing problems.
Exploratory data analysis:
Exploratory analysis techniques are used in EDA to study, examine, and analyze the set to identify its properties. To obtain the answers, it best manipulates the data.
Model building and deployment:
We will utilize data modeling, machine learning, statistical models, and algorithms to gather data from the process and create predictions. Although deployment is the final step in the CRISP-DM methodology, your project may still need to be finished at that point. The planning and recording of the model’s deployment and the delivery and presentation of the results occur during the deployment phase. You’ll also need to maintain the model and monitor the results during deployment.
Conclusion:
In this blog, you would have understood Data Science and its steps. To learn more about Data Science, Join Data Science Courses in Bangalore, where our expert trainers will train you with real-time examples and case studies.